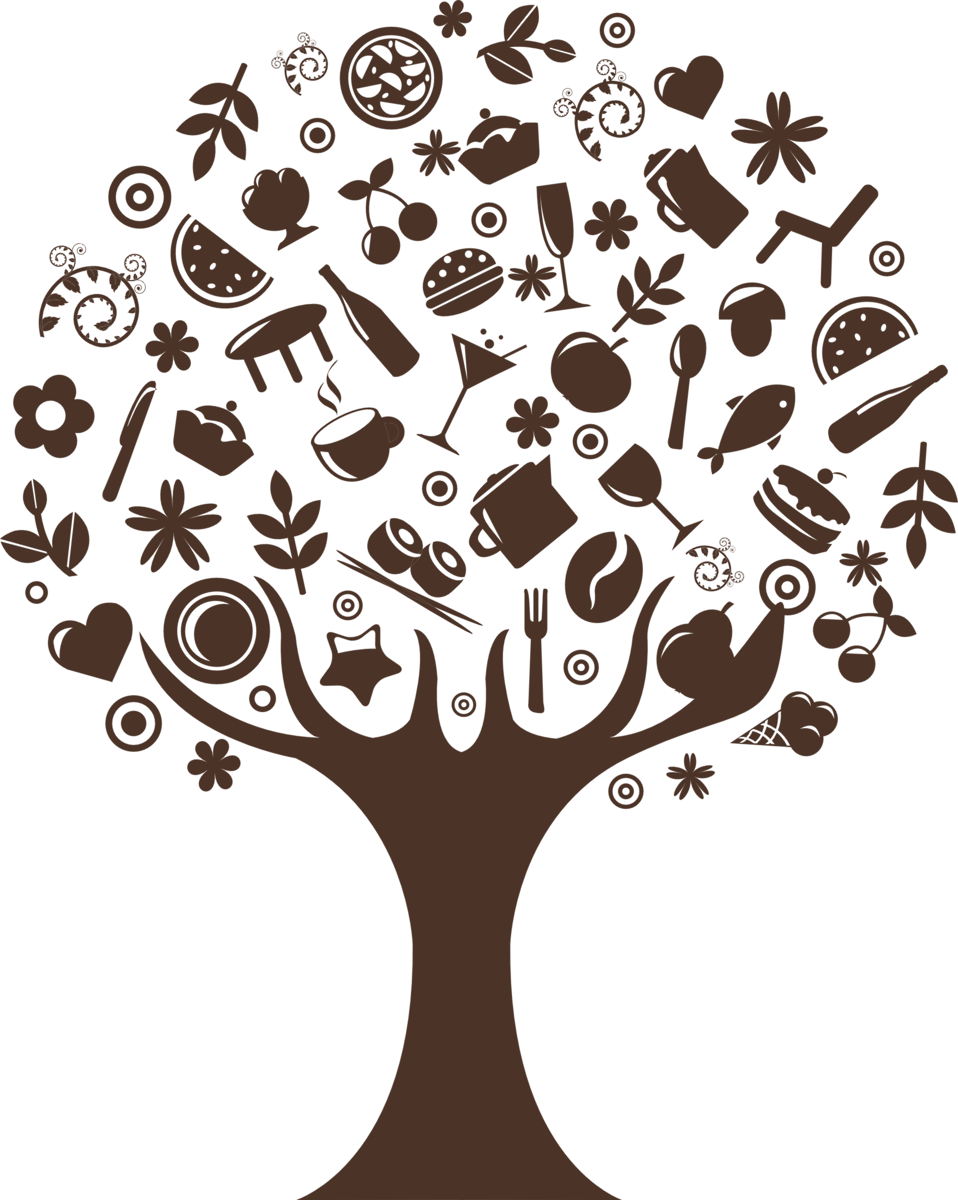
Other Research
In our lab, we are open to conducting a range of research that doesn’t fit into our otherwise broad portfolio. Here you will find information about publications from these projects.
Blockchain
Bitcoin’s success has led to significant interest in its underlying components, particularly blockchain technology. Over 10 years after Bitcoin’s initial release, the community still suffers from a lack of clarity regarding what properties defines blockchain technology, its relationship to similar technologies, and which of its proposed use-cases are tenable and which are little more than hype. In our research,1 we have answered four common questions regarding blockchain technology:
- What exactly is blockchain technology?
- What capabilities does it provide?
- What are good applications for blockchain technology?
- How does it relate to other distributed technologies (e.g., distributed databases)?
Our finding show that Blockchain technology is most appropriate under three conditions: (a) a need for shared governance and operation (i.e., not trusted central parties can conduct any of these responsibilities), (b) auditable state, and (c) resilience to data loss.
We are currently exploring applications for blockchain technology in two areas. First, distributed document management for health care. Second, supporting multi-organizational humanitarian aid and disaster relief. In both these situations, there is no central authority to handle governance and operation, but there is a need for organizations to work with each other. Our research aims to build tools that will support both of these use cases, including in situations with limited Internet connectivity—situations which are critical for both applications, but which are not currently well-supported by existing blockchain techniques.